In today’s rapidly advancing world of artificial intelligence (AI), the pace of innovation is both exhilarating and relentless. As AI continues to evolve, it’s not enough for companies to simply keep up with emerging technologies—they must actively lead the charge, continually seeking out and mastering the most advanced tools available. This is especially true in a landscape where the demands for smarter, more efficient, and contextually aware systems are higher than ever. The ability to harness the full potential of these cutting-edge technologies is what distinguishes industry leaders from the rest. It’s about more than just adopting the latest innovations; it’s about integrating them into real-world solutions that deliver tangible value, whether through improved user experiences, enhanced regulatory compliance, or optimized data management.
One of the most promising technologies to emerge in recent years is Retrieval-Augmented Generation (RAG), a sophisticated AI architecture that marries the best of both retrieval-based and generative models. By combining these two approaches, RAG allows for the creation of AI systems that are not only intelligent but also remarkably accurate and contextually relevant. This dual capability is what sets RAG apart from traditional AI models, making it a transformative force in the field. At Simelabs, we’ve recognized the transformative potential of RAG early on and have been at the forefront of its implementation across various projects. Our commitment to innovation has driven us to explore how RAG can be applied to enhance user interactions, streamline complex regulatory processes, and improve the accessibility and accuracy of data. By leveraging RAG, we’re not just responding to the challenges of today’s AI landscape—we’re setting new standards for what’s possible, pushing the boundaries of AI to create solutions that are smarter, more efficient, and more aligned with the needs of our clients.
What is Retrieval-Augmented Generation (RAG)?
RAG is an AI architecture that combines the strengths of retrieval-based systems with generative models to provide highly accurate, contextually relevant responses. The concept revolves around integrating a retrieval mechanism that pulls in relevant information from a large dataset or knowledge base and feeding it into a generative model like GPT (Generative Pre-trained Transformer). This architecture is particularly effective in scenarios where the accuracy of information and context is paramount.
Traditional generative models, while powerful, sometimes struggle with factual accuracy, especially when dealing with specialized or domain-specific knowledge. RAG addresses this by first retrieving the most pertinent data and then using this data to generate responses, ensuring that the output is both contextually aware and factually correct.
Case Study 1 : Streamlining Regulatory Compliance with RAG
Navigating regulatory compliance in the pharmaceutical industry presents a unique set of challenges. The process is often intricate and labor-intensive due to the varying timelines, procedures, and requirements imposed by different countries. Recognizing the need for a more efficient approach, Simelabs developed a solution utilizing Retrieval-Augmented Generation (RAG) to automate and streamline the reporting of Individual Case Safety Reports (ICSRs) and other safety information.
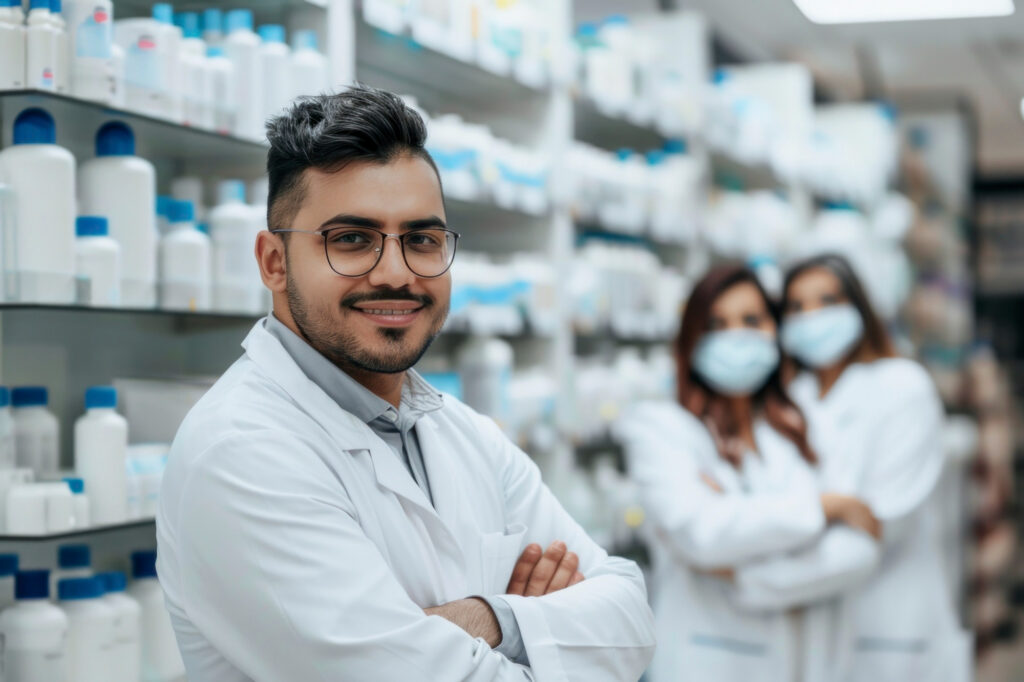
Challenges
The pharmaceutical sector faces a myriad of regulatory hurdles, largely driven by the diverse requirements that differ from one country to another. These requirements can vary based on several factors, including the stage of the product (pre-marketing or post-marketing), the type of product (drugs, vaccines, biologics, etc.), and the nature of the reporting (expedited, periodic, or risk management). Additionally, pharmaceutical companies must adhere to strict timelines for report submissions as stipulated by regulatory bodies, adding to the complexity of compliance.
Benefits
The implementation of RAG in our regulatory compliance solution brings several key benefits. The automation of data collection and structuring significantly reduces manual effort and minimizes the potential for errors, enhancing overall efficiency. By ensuring that companies meet regulatory requirements across different countries and product types, our solution supports rigorous adherence to compliance standards. Moreover, the provision of data through a REST API makes critical regulatory information readily accessible, enabling pharmaceutical companies to maintain compliance effortlessly. Through the application of RAG, Simelabs has successfully streamlined the regulatory compliance process, offering a solution that meets the complex demands of the pharmaceutical industry with greater precision and ease.
Case Study 2 : CartGPT – Revolutionizing E-commerce with RAG
At Simelabs, our commitment to pushing the boundaries of AI technology is exemplified in our flagship project, CartGPT. This sophisticated chatbot represents a transformative application of Retrieval-Augmented Generation (RAG) within the e-commerce sector. Built using OpenAI models and leveraging the power of RAG, CartGPT is designed to provide an unparalleled user experience by seamlessly interacting with product catalogs.
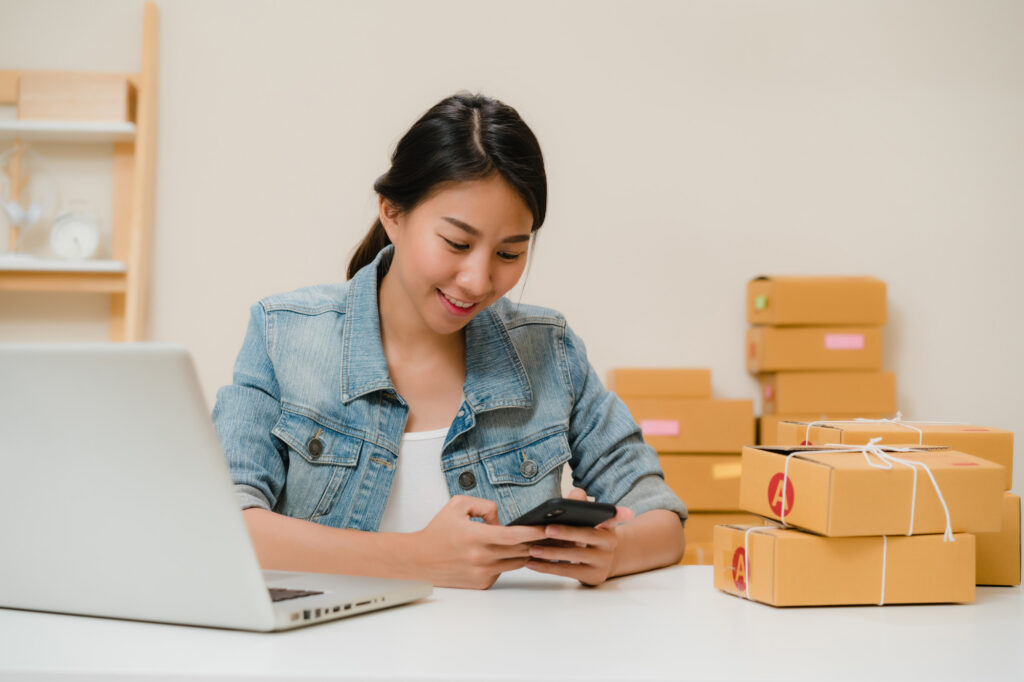
Advanced Search Capabilities
CartGPT stands out for its advanced search capabilities, which are central to its functionality. The chatbot is capable of handling a diverse range of search types, enabling users to find exactly what they need with remarkable speed and precision. This functionality is a direct result of RAG’s ability to retrieve and present the most relevant information from a vast product catalog, enhancing the efficiency of the search process.
Contextual Product Suggestions
One of the key strengths of CartGPT is its ability to provide contextual product suggestions. By deeply understanding user queries through RAG, CartGPT can offer product recommendations that are highly relevant to individual needs. This capability not only improves the accuracy of the suggestions but also enriches the overall shopping experience, making it more personalized and engaging for users.
Accurate Information Retrieval
Accuracy in information retrieval is a crucial aspect of CartGPT’s functionality. By leveraging RAG, the chatbot ensures that every response is both accurate and contextually relevant. The RAG architecture allows CartGPT to draw from an extensive dataset within the product catalog, ensuring that users receive reliable information in real-time.
Impact
The implementation of CartGPT has significantly transformed user interactions with e-commerce platforms. The chatbot’s advanced capabilities, powered by RAG, enable it to not only understand but also anticipate user needs. This results in a more intuitive and enjoyable shopping experience, where users can interact with the platform in a way that feels both natural and intelligent. CartGPT’s success highlights Simelabs’ expertise in applying RAG to enhance AI-driven solutions, setting a new standard for e-commerce interactions.
Through CartGPT, Simelabs has demonstrated the transformative potential of RAG, showcasing how this technology can revolutionize user experiences and deliver significant advancements in the e-commerce sector.